Call for Papers
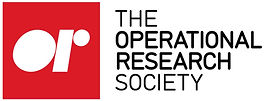
Journal of
Business Analytics
Generative AI models: challenges and opportunities for business analytics
Extended Deadline: 30th September 2024
Guest editors
-
Dursun Delen, Oklahoma State University, USA. Email: dursun.delen@okstate.edu
-
Benjamin George, University of Toledo, USA. Email: benjamin.george3@utoledo.edu
-
Ashish Gupta, Auburn University, USA. Email: azg0074@auburn.edu
-
Samuel Kirshner, University of New South Wales, Australia. Email: s.kirshner@unsw.edu.au
-
Martin Kunc, University of Southampton, UK. Email: M.H.Kunc@soton.ac.uk
-
Michael Mortenson, University of Warwick, UK. Email: Michael.Mortenson@wbs.ac.uk
-
David Olson, University of Nebraska–Lincoln, UK. Email: david.olson@unl.edu
-
Sudha Ram, University of Arizona, USA. Email: ram@eller.arizona.edu
-
Raha Akhavan Tabatabaei, Sabancı University, Turkey. Email: akhavan@sabanciuniv.edu
-
Richard Vidgen, University of New South Wales, Australia. Email: r.vidgen@unsw.edu.au
-
Huimin Zhao, University of Wisconsin-Milwaukee, USA. Email: hzhao@uwm.edu
Timeline
-
Submission due date: 30 September 2024
Background
Artificial Intelligence (AI) has emerged as a disruptive force, transforming industries, reshaping our lives and work, and significantly impacting businesses, institutions, and societies. Driven by advancements in machine learning and deep learning, as well as the exponential growth of available data, AI technologies have enabled machines to handle complex tasks such as natural language processing, image recognition, and automated decision-making (Brynjolfsson & McAfee, 2014; Jordan & Mitchell, 2015; Shollo et al., 2022).
AI’s rapid evolution has led to its widespread adoption, with applications ranging from automating mundane tasks to enhancing productivity and enabling new forms of human-computer collaboration across various sectors, including healthcare, finance, manufacturing, retailing, and education (Dwivedi et al., 2021). The technology offers significant potential for businesses and society by improving efficiency, reducing costs, and providing better and faster decision-making capabilities (Dwivedi et al., 2023).
Recent developments in Generative AI and large language models (LLMs), such as OpenAI's GPT-3, GPT-4, and ChatGPT, have expanded AI’s capabilities and accessibility. These models use deep learning techniques to “understand” and generate human-like responses in natural language (Lund et al., 2023), with applications in virtual assistants, content generation, and sentiment analysis (Radford et al., 2019; Dwivedi et al., 2023). Further developments in AI include reinforcement learning and generative adversarial networks (GANs). We refer to these technologies collectively as Generative AI (GenAI).
The impact of GenAI on organisations and societies can be both positive and negative. Organisational concerns include job displacement, increased dependency on technology, and potential biases in decision-making due to flawed algorithms (O'Neil, 2017; Marjanovic et al., 2021). Societal risks involve privacy invasion, manipulation of public opinion, and widening socio-economic disparities due to unequal access to AI technologies.
Objective of the special issue
The objective of this special issue is to publish papers that contribute to both the theory and practice of GenAI in business analytics.
We welcome submissions that show how GenAI can be applied in practice with consideration of value creation, value destruction, and safeguarding for citizens, communities, organizations, society at large, and the natural environment. We further welcome papers that consider how these various stakeholders can respond to, manage, and cope with change associated with GenAI.
Specifically, this special issue aims to invite business analytics scholars and practitioners to look at GenAI technologies with respect to:
-
Current and potential implications of GenAI on business operations and supply chains
-
Application of GenAI in marketing and consumer behavior
-
Customer service and support and chatbots
-
Potential applications of GenAI in healthcare including mental health
-
Potential benefits and pitfalls of GenAI in human resources
-
GenAI and the future of work (job creator and job taker)
-
Impact of GenAI to the work of executives and business managers
-
Impact of GenAI on the role of the business analytics professional
-
Impact of GenAI to task automation; is there a safe boundary?
-
ChatGPT-type technologies for information retrieval and question answering, content creation and writing assistance, multilingual communication and translation
-
Conversational ChatGPT applications in organizations
-
GenAI and accessibility and inclusive technology
-
Ethics, policy, and governance issues with GenAI
-
Organizational and social issues in GenAI adoption
-
GenAI applications in, and impact on, education
-
Societal and economic impacts of GenAI including the democratic process, justice, digital divide, and fairness
-
Natural environmental and climate change impacts of AI
-
GenAI in the metaverse and gaming
-
GenAI and existential risk (e.g., for workers, professions, organizations, society, and humanity)
Articles on other topics that have relevance for GenAI and business analytics are also welcome.
Article types
We seek submissions in the following forms:
-
Research papers
-
Industry reports (we particularly welcome collaborations between industry practitioners and academics)
-
Viewpoint articles
References
Brynjolfsson, E. & Mcafee, A. (2014). The second machine age: Work, progress, and prosperity in a time of brilliant technologies, WW Norton & Company.
Dwivedi, Y. K., Hughes, L., Ismagilova, E., Aarts, G., Coombs, C., Crick, T., ... & Williams, M. D. (2021). Artificial Intelligence (AI): Multidisciplinary perspectives on emerging challenges, opportunities, and agenda for research, practice and policy. International Journal of Information Management, 57, 101994. https://www.sciencedirect.com/science/article/pii/S026840121930917X
Dwivedi, Y. K., Kshetri, N., Hughes, L., Slade, E. L., Jeyaraj, A., Kar, A. K., ...& Wright, R. (2023). “So what if ChatGPT wrote it?” Multidisciplinary perspectives on opportunities, challenges and implications of generative conversational AI for research, practice and policy. International Journal of Information Management, 71, 102642.
Jordan, M. I. & Mitchell, T. M. (2015). Machine learning: Trends, perspectives, and prospects. Science, 349, pp. 255-260.
Lund, B. D., Wang, T., Mannuru, N. R., Nie, B., Shimray, S. & Wang, Z. (2023). ChatGPT and a new academic reality: Artificial Intelligence‐written research papers and the ethics of the large language models in scholarly publishing. Journal of the Association for Information Science and Technology, 74, pp. 570-581.
Marjanovic, O., Cecez-Kecmanovic, D., and Vidgen, R., (2021). Algorithmic pollution: making the invisible visible. Journal of Information Technology, 36(4): 391–408.
O'Neil, C. (2017). Weapons of math destruction: How big data increases inequality and threatens democracy, Crown.
Shollo, A., Hopf, K., Thiess, T. & Müller, O. (2022). Shifting ML value creation mechanisms: A process model of ML value creation. The Journal of Strategic Information Systems, 31, p. 101734.