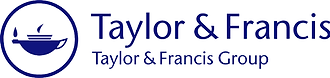
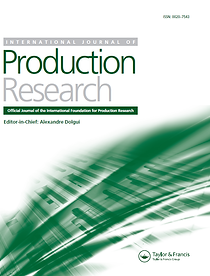
International Journal of Production Research
Digital Twin Transition for Intelligent and Resilient Industrial Systems
Guest editors
-
Serena Finco, University of Padova, Italy. Email: serena.finco@unipd.it
-
Jinsong Bao, Donghua University, China
-
Ilya Jackson, MIT, USA
-
Yuqian Lu, University of Auckland, New Zealand
Submission timeline and instructions
-
Deadline for manuscript submission: 25 January 2025
Please select the special issue option "Digital Twin Transition for Intelligent and Resilient Industrial Systems" on the submission form.
About the issue
Nowadays, digitalization represents a crucial driver in achieving higher levels of productivity, resilience, sustainability and flexibility for all production, manufacturing and logistics systems as well as supply chains (Lu et al, 2020). Digital technologies, such as Internet of Things, Cloud Computing, Artificial Intelligence, Extended Reality or the new generation of Information Technologies, allow an intelligent integration and interconnection among all actors involved in the processes of each manufacturing system. Moreover, digital technologies enable real-time monitoring, control, and data collection, as well as the development of cyber-physical systems that are able to combine physical and virtual environments. In such a context, the Digital-Twin (DT) concept has gained ground in the last decade, and they are widely used during the design and operational decision processes. Several states of the art confirm the increasing importance of digitalization (Psarommatis et al., 2022; Semeraro et al., 2021; Lu et al., 2020; Kritzinger et al., 2018). DT is defined in several ways according to the application field.
DT is designed to continuously process data from the past, monitor the present in real-time and, finally, support proactive decision-making. DT is applied during design and operational decisions such as production planning and control, workpiece quality prediction, machine and human-robot collaboration, real-time monitoring, product traceability, performance prediction (Luo et al., 2022; Wang et al., 2021). Recently, due to the new Industry 5.0 era, the concept of Human DT has born (Bilberg et al., 2019). In such a scenario, digitalization tools help designers, managers and academics in detecting, collecting, improving and providing info about the health status, the mental or physical fatigue level, the posture of workers involved in the production or logistics processes (Lu et al., 2021; Lu et al., 2022). In such a way, the self-resilient skilled operator concept, which is the main pilar of Industry 5.0, can be easily achieved (Romero, 2021).
The concept of DT for supply chain is defined by Ivanov (2023) as a virtual system consisting in three elements: 1) digital visualization 2) digital technologies and 3) descriptive, predictive and prescriptive analytics for decision making support. In such a context, DT can be used to detect disruption, improve resilience, analyze sustainability aspects, create efficient demand planning and monitor inventory and shipment.
Some criticalities exist in implementing DT. For example, there are difficulties in sharing DT in multiple application systems involving several stakeholders, difficulties in ensuring efficient storage, processing and analysis of a large amount of data (Psarommatis, 2022). Furthermore, there is the necessity to ensure reliability and robustness of the DT, and, due to the high degree of heterogeneity in digital technologies, there are some difficulties in making suitable decisions and investments in DT from a company perspective. Additionally, it is necessary, especially for small-medium companies, to understand the implementation costs and the return of investment for all technologies leading to a DT environment. Finally, there are still some gaps related, for example, to complex manufacturing systems and external and internal factors that can affect the degradation of machines or workers' skills.
For, this reason, this special issue aims to close some gaps related to DT in production systems and supply chain.
Topics
We invite papers that address, but are not limited to, the following topics:
-
Using new emerging technologies for the Digital Twin implementation in industrial systems
-
Conceptual frameworks to support Digital Twin development in production and logistics systems
-
Digital Twin for sustainable manufacturing and distribution systems
-
Real-time-based models and algorithms for assembly line design, balancing, and rebalancing techniques
-
Simulation and optimization models based on real-time data for production planning and control in flexible manufacturing systems
-
Real-Time dynamic job scheduling and sequencing for complex manufacturing systems
-
Digital Twin models for maintenance
-
Digital Twin models for analysing the Machine-to-Machine interaction and Human-Robot collaboration
-
Human Digital Twin for improving ergonomics and workers’ well-being
-
Digital twin to improve reliability, availability, and efficiency
-
Digital twin as a tool to improve production resilience
-
Digital twin in operations and supply chain management
-
Digital twin for supply chain resilience
-
Digital thread in supply chain
-
Quantitative and qualitative analysis of the implementation of Digital twin
-
Economic analysis (e.g., ROI, costs-benefits analysis) for implementing digital twin
-
Digital twin applications in real manufacturing systems
Both theoretical and applied research contributions and real-world application feedback are welcome. All papers should adhere to the IJPR scope and publication criteria.
References
-
Bilberg, A., & Malik, A. A. (2019). Digital twin driven human–robot collaborative assembly. CIRP annals, 68(1), 499-502.
-
Ivanov, D. (2023). Conceptualisation of a 7-element digital twin framework in supply chain and operations management. International Journal of Production Research, 1-13.
-
Kritzinger, W., Karner, M., Traar, G., Henjes, J., & Sihn, W. (2018). Digital Twin in manufacturing: A categorical literature review and classification. Ifac-PapersOnline, 51(11), 1016-1022.
-
Kusiak, A. (2022). Predictive models in digital manufacturing: research, applications, and future outlook. International journal of production research, 1-11.
-
Lu, Y., Adrados, J. S., Chand, S. S., & Wang, L. (2021). Humans are not machines—anthropocentric human–machine symbiosis for ultra-flexible smart manufacturing. Engineering, 7(6), 734-737.
-
Lu, Y., Liu, C., Kevin, I., Wang, K., Huang, H., & Xu, X. (2020). Digital Twin-driven smart manufacturing: Connotation, reference model, applications and research issues. Robotics and computer-integrated manufacturing, 61, 101837.
-
Lu, Y., Zheng, H., Chand, S., Xia, W., Liu, Z., Xu, X., ... & Bao, J. (2022). Outlook on human-centric manufacturing towards Industry 5.0. Journal of Manufacturing Systems, 62, 612-627.
-
Luo, D., Thevenin, S., & Dolgui, A. (2022). A state-of-the-art on production planning in Industry 4.0. International Journal of Production Research, 1-31.
-
Psarommatis, F., & May, G. (2022). A literature review and design methodology for digital twins in the era of zero defect manufacturing. International Journal of Production Research, 1-21.
-
Psarommatis, F., & May, G. (2022). A standardized approach for measuring the performance and flexibility of digital twins. International Journal of Production Research, 1-16.
-
Romero, D., & Stahre, J. (2021). Towards the resilient operator 5.0: The future of work in smart resilient manufacturing systems. Procedia CIRP, 104, 1089-1094.
-
Semeraro, C., Lezoche, M., Panetto, H., & Dassisti, M. (2021). Digital twin paradigm: A systematic literature review. Computers in Industry, 130, 103469.
-
Wang, K. J., Lee, Y. H., & Angelica, S. (2021). Digital twin design for real-time monitoring–a case study of die cutting machine. International Journal of Production Research, 59(21), 6471-6485.